Every business is on the clock to figure out their “AI strategy.” Educators, too.
Helping companies surf the latest technological waves is nothing new to Stanford professor Gabriel Weintraub, who has advised Airbnb, Outschool and others on matters of data science, marketplaces and management science.
Currently the Amman Mineral Professor of Operations, Information & Technology at Stanford Graduate School of Business, Weintraub closely studies the impact of technology on economics, operations and digital platforms. He is also an advisor to our AI + Catalyst, our pre-seed program for early-stage AI edtech companies.
We recently caught up with him for his thoughts on how startup founders can adapt and capitalize on the latest technology breakthrough.
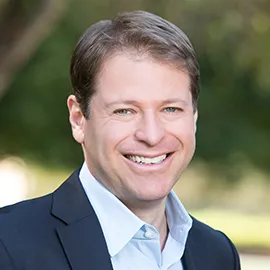
Gabriel Weintraub, Amman Mineral Professor of Operations, Information & Technology at Stanford Graduate School of Business
There’s a lot of emphasis on finding an “AI strategy.” What are the most important elements to consider?
With any technology hype cycle, it’s easy to fall in love with the tech and treat it as a hammer. Everything is a nail, and you want to use it everywhere. Don’t lose sight of the fact that as a business you’re supposed to be solving a problem and pain point for your customers. Successful entrepreneurs fall in love with the problem, not the tech. This is the starting point.
AI is changing very fast, but fundamental business principles haven’t. Your focus is on creating value, and AI tools offer new ways to create value through product enhancements, improving cost structures and streamlining your workflows and processes. To do so, you must thoughtfully match AI strength with your business objectives.
Of course creating value is not enough: you also need ways of capturing that value in this AI environment. Owning user interactions, proprietary data, domain knowledge, and building your own AI models can enable value capture.
Building on your experience in data science, how does AI change this role and function?
I foresee two significant transformations that AI can have on data science (DS) teams. First, the advent of AI may simplify the creation of self-serving tools. With users employing natural language processing to locate and analyze data, routine demands on DS teams to do these tasks can be reduced. This can foster a more user-centric and intuitive approach to data exploration.
Second, AI has the potential to automate the frequently repetitive tasks like cleaning and structuring data, along with other workflows. This enhances efficiency and productivity within the DS team. Similarly with building models and coding. These AI-enabled changes can liberate data scientists from more routine tasks so that they can invest their time on the more impactful and creative aspects of their work. Such a shift in focus could catalyze even greater innovation and value creation.
What are common challenges for online marketplace businesses (particularly in education) that AI can help companies address?
Online marketplaces were created to reduce frictions such as those associated with search and information. AI can reduce such frictions and enhance the efficiency and quality of matches and improve users’ overall experiences. This typically necessitates preference elicitation, a process that can range from straightforward (like purchasing a product in e-commerce) to complex (such as booking a vacation).
AI can significantly streamline and simplify this process through natural language. Imagine planning a trip: Instead of navigating through multiple filters, options, and webpages, you could simply describe your ideal vacation. Whether you are planning a beach family vacation or a solo hiking expedition, the AI could understand your preferences and provide tailor-made suggestions, which could drastically simplify the search and matching process.
AI can also help automate the processing of unstructured data (from text, pictures or videos) to assess the quality of products and services. This will help enhance curation on the supply side of marketplaces. A real game-changer will be the creation of hyper-personalized experiences and pathways. By learning from each interaction, AI can curate recommendations that align closely with individual tastes and preferences, making the user experience more personal and engaging, which in turn can create more business opportunities for suppliers.
Where do you see the startups having an advantage over incumbent, bigger companies with established distribution channels and data?
Big companies can suffer from inertia. For those that are not AI-native, it may take considerable effort to deal with the technical debt and maybe even redo the tech stack to integrate the latest AI tools. Companies that pivot quickly are more likely to succeed in this environment and their current distribution channels and data gives them an advantage. Speed, flexibility and adaptability are important advantages, especially at a time when the technology is evolving so fast.
Barriers to entry have gone down with the new AI tools at our disposal. Where it once took 10 developers to build a product, AI tools like Github Copilot can help you do it with half the number, maybe even fewer. They make it easier to test and refine your products, and speed up the data collection and feedback loop so that they can improve products and data models. All of this can facilitate competing with established incumbents.
What new risks posed by AI should we be mindful of?
AI presents significant potential risk particularly for spreading misinformation and affecting the job market that have been widely discussed. An overlooked area, from a data science perspective, are AI’s own weaknesses when it comes to bias, hallucination, its probabilistic nature, and potential for errors.
Previously, most of the effort developing machine learning and AI models went into the data inputs. The old saying “garbage in, garbage out” was a guiding principle. But it’s increasingly clear that we need to focus more on the outputs to mitigate the risks mentioned above. This shift means we’ll have to invest more in evaluation, testing, and monitoring models. Paying careful attention to edge cases and biases is especially important now, as these AI models aren’t just making predictions anymore. They’re also playing a critical, sometimes decisive role in interactions with users, students, and patients.
What are the most exciting applications of AI today?
Education and health are the areas that I’m most excited about. I’m in Chile right now, and in rural schools resources are incredibly constrained — especially teachers. There may just be only one teacher for the entire elementary school. Every kid has potential, but not every kid has a fair chance at the opportunity to learn and develop that potential. I see a huge AI opportunity here to provide access to higher quality education specially for underserved populations.
For example, an AI-powered platform could supplement conventional classroom teaching, providing personalized learning pathways for each student. It could help in recognizing a student’s strengths and areas for improvement, adapt to their learning pace, and offer targeted, engaging educational content. This approach could, to a certain extent, compensate for the shortage of teachers.
Hence, figuring out how you pair teachers with the latest AI technology is critical. It’s a fine balance and there won’t be one simple answer that works for everybody, but there is tremendous potential and it’s important that we continue to experiment and iterate.
How are you using it in your classes?
AI will certainly change what we teach, how we teach, and how we evaluate students. There is huge potential as well as important challenges. It’s a work in progress and we’re still figuring it out.
It’s already happening where people in certain workplaces will be expected to use these tools to code, to write, et cetera. So in the end, you must teach them how to operate these tools. Knowing how to use Copilot as a coding assistant is part of the required skill set for some companies looking to hire developers.
Still, we may need to restrict the use of these tools for children and even students in undergrad and grad programs, so that they develop the basic knowledge and technical skills needed to make the most of AI tools in their future. We want them to be effective yet critical users of these tools.
At a more concrete level, one promising impact will be to automate repetitive tasks such as grading for professors and teaching assistants. If AI can assist with that, the teaching team can focus on sparking more creative, meaningful discussions than spending so much time on mundane tasks.
This is only the beginning. How AI shapes the human teaching and learning processes will require careful thought, and a lot of iteration.